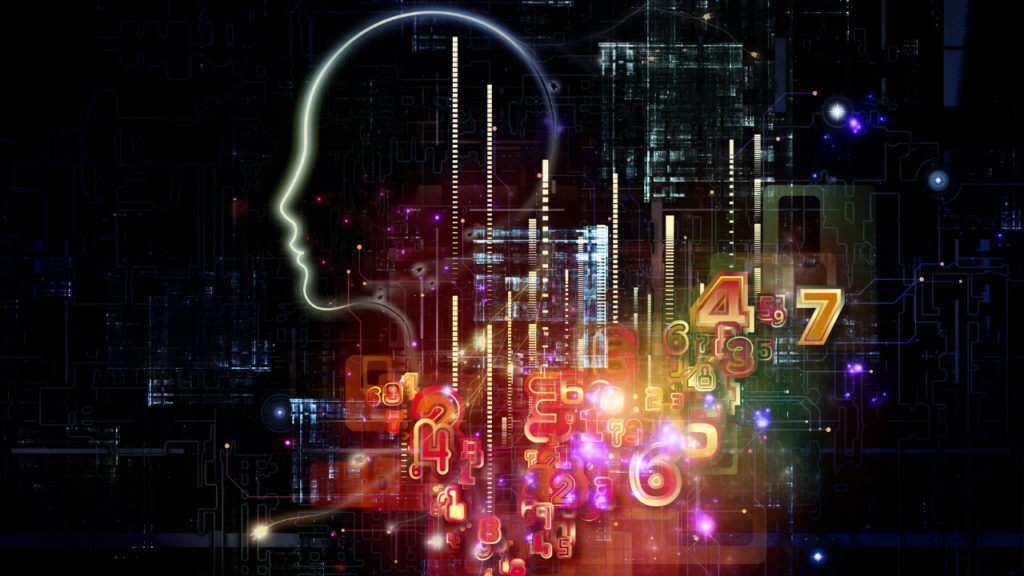
Conversational Intelligence: When Good Intentions Meet Bad Data
By: Wayne Lopez, Chief Product Officer at Vertify
Conversational Intelligence has emerged as a powerful framework for improving communication and building trust in personal and professional interactions. Proponents of Conversational Intelligence emphasize the ability to understand and navigate the neurological underpinnings of communication, fostering positive and productive conversations. However, a critical examination reveals a potential pitfall: the reliance on incomplete or inaccurate data can lead to unintended consequences and undermine the very goals of Conversational Intelligence.
Conversational Intelligence holds immense promise in B2B sales and marketing, aiming to improve customer engagement and lead generation. However, its reliance on data can lead to missteps in this complex environment.
Laying the Foundation for Better Understanding
One key area of concern lies in the oversimplification of complex human behavior. Conversational Intelligence frameworks often categorize behavior into distinct “levels” based on specific observable actions. While this can offer a starting point for understanding, it risks overlooking the nuance and context that shape individuals’ communication styles. Factors like cultural background, personal experiences, and emotional states can significantly influence how someone expresses themselves. Over reliance on rigid categories can lead to misinterpretations and misjudgments, ultimately hindering the creation of genuine connections.
Furthermore, Conversational Intelligence heavily emphasizes the neuroscientific basis of communication. While understanding the brain’s role in communication is valuable, it’s crucial to acknowledge the limitations of this data. Neuroscience is still a rapidly evolving field, and current knowledge does not provide a complete picture of the intricate dance between thoughts, emotions, and behavior. Basing communication strategies solely on this incomplete picture can lead to a reductionist approach, neglecting the holistic nature of human interaction.
Moreover, the emphasis on specific techniques within Conversational Intelligence frameworks can inadvertently create a formulaic approach to communication. While specific tools like active listening can be valuable, focusing solely on their application can lead to inauthentic interactions. Authentic communication thrives on flexibility and genuine connection, not the rigid application of pre-defined techniques.
So, what can be done to address these challenges? Here are a few key takeaways:
- Embrace the complexity: Recognize that human communication is multifaceted and influenced by a myriad of factors.
- Context is king: Always consider the context of the situation and the individuals involved before applying any framework or technique.
- Focus on genuineness: Prioritize building genuine connections and understanding that goes beyond specific methods.
- Remember, data is not destiny: Use data as a guide, not a gospel, and constantly seek to broaden your understanding of human interaction.
It’s Not That Simple for B2B Businesses
But, the plot thickens…
Let’s specifically hone in on Conversational Intelligence for B2B businesses. Here’s what it might get wrong in the B2B world:
- Overlooking Industry Specificity: Conversational Intelligence frameworks often focus on generic communication principles, neglecting the unique nuances of specific industries. B2B sales often involve technical jargon, regulatory hurdles, and complex decision-making processes. A one-size-fits-all approach based on generic data may not capture these crucial industry-specific nuances, leading to misinterpretations and ineffective communication.
- Underestimating the Power of Relationships: Building strong relationships is paramount in B2B sales. Conversational Intelligence, however, might prioritize data-driven tactics over understanding the human element of building trust and rapport. This can result in inauthentic and transactional interactions, hindering the development of long-term partnerships.
- Misinterpreting Customer Data: Conversational Intelligence tools often analyze past interactions to understand customer behavior. However, relying solely on historical data can overlook evolving customer needs and market trends. Additionally, focusing only on quantitative data (e.g., buying patterns) may miss crucial qualitative aspects (e.g., customer pain points, aspirations) that are vital for crafting compelling messaging.
- Difficulty Navigating Power Dynamics: B2B sales often involves negotiating contracts and navigating power dynamics. Conversational Intelligence frameworks may lack the specific guidance needed to navigate these situations effectively. Relying solely on data analysis could lead to a blunt and insensitive approach that alienates potential customers.
- Neglecting the Importance of Creativity: While data-driven insights are valuable, creativity plays a crucial role in crafting unique and compelling B2B marketing campaigns. Relying heavily on data-driven analysis could stifle creative thinking, leading to generic and forgettable messaging that fails to resonate with target audiences.
Best-of-Breed Data Management Practices Can Save the Day
Data management is a powerful weapon against the pitfalls of bad data in Conversational Intelligence. Here’s how:
1. Ensuring Data Quality and Consistency:
- Standardization: Implement consistent data formats across all sources, ensuring information compatibility and reducing the risk of misinterpretations during analysis.
- Data Cleansing and Validation: Regularly evaluate data for errors, inconsistencies, and missing values. Cleanse and correct any discrepancies to ensure the accuracy and reliability of the information used by intelligence tools.
- Data Profiling: Analyze data to understand its structure, distribution, and potential biases. Identify and address any biases to prevent their influence on insights and recommendations.
2. Prioritizing Data Relevancy and Context:
- Data Mapping: Connect data points from various sources to build a complete picture of the conversation and the individuals involved. This helps Conversational Intelligence tools understand the context surrounding the data for more accurate interpretations.
- Segmentation and Targeting: Segment data based on relevant factors like customer type, industry, or product interest. This allows intelligence systems to deliver contextually relevant insights and recommendations tailored to specific scenarios.
- Data Governance: Establish clear guidelines and procedures for data collection, storage, and access. This ensures the responsible use of data and prevents the misuse of personal information.
3. Emphasizing the Human Element:
- Human-in-the-Loop Approach: Combine data analysis with human expertise. Leverage the knowledge and experience of professionals who understand the nuances of human interaction to interpret data and apply insights effectively.
- Qualitative Data Integration: Include qualitative data like customer feedback, sentiment analysis, and call transcripts alongside quantitative data. This provides a more comprehensive view of customer interactions and their emotions, offering a deeper understanding than just raw data analysis alone.
4. Continuous Monitoring and Improvement:
- Data Lineage and Auditing: Track the origin and transformation of data used in Conversational Intelligence tools. This ensures traceability and facilitates the identification and correction of any errors that may arise during the process.
- Regular Reviews and Updates: Regularly review and update data management practices to ensure they remain aligned with evolving business needs and regulatory requirements.
- Feedback Loop: Establish a feedback loop where insights from intelligence tools are used to improve data collection, analysis, and interpretation processes.
Final Thoughts
By adhering to these best practices, organizations can ensure that their data is accurate, relevant, and contextual, allowing intelligence tools to provide valuable insights that enhance – rather than hinder – communication effectiveness. Remember, data is only as good as the practices used to manage it. By prioritizing data quality and combining it with human expertise and qualitative information, organizations can unlock the true potential of Conversational Intelligence and navigate conversations with confidence.
In addition, by acknowledging the limitations of data and fostering a more holistic approach to communication, you can ensure that Conversational Intelligence truly lives up to its potential of fostering meaningful connections and positive outcomes.
Remember, the goal is to enhance communication, not to turn it into a science experiment. Harness the combined power of Conversational Intelligence and best-of-breed data management practices to bridge the gap between good intentions and successful interactions, one authentic conversation at a time.
Vertify is the data automation platform that lets you customize workflows, anyway you want. It works beautifully with revenue apps and intelligence platforms to deliver trusted customer and prospect data into the hands of sales and marketing people across the globe. Are you ready to take the plunge? Click here to connect with Vertify today!